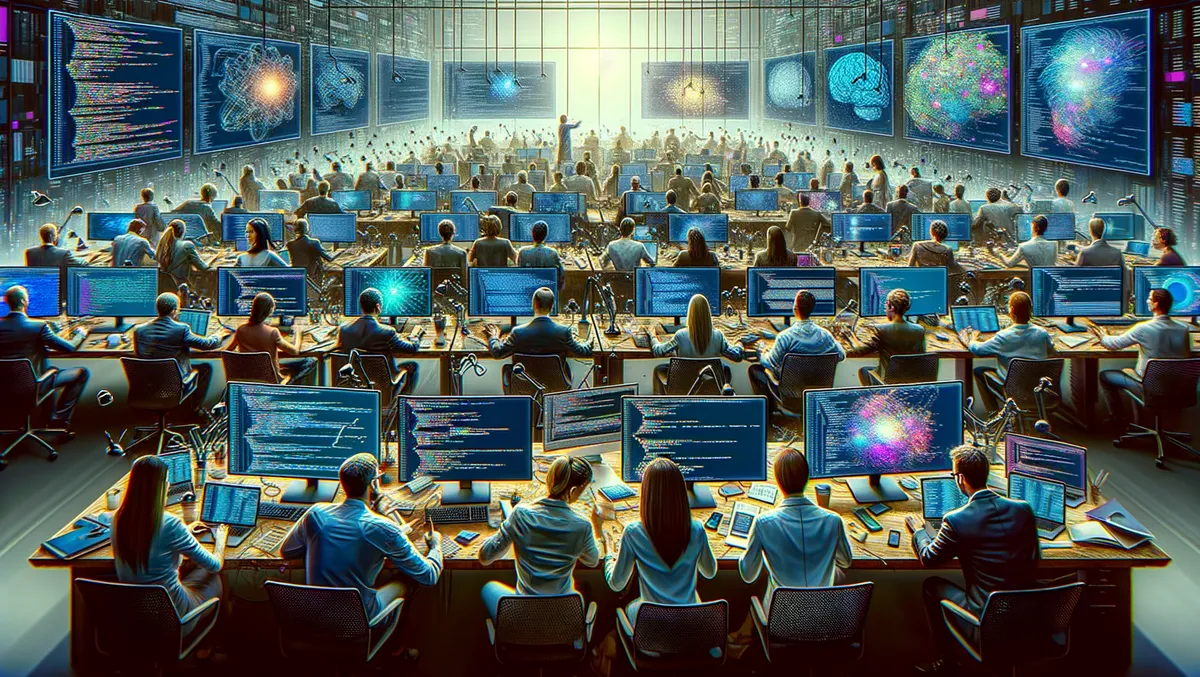
Half of machine learning developers find projects time-consuming
A recent survey carried out by Civo, the sole pure-play cloud-native service provider, discovered that a significant 48% of machine learning (ML) developers consider ML projects to be too time-consuming. The study, which involved more than 500 ML developers, revealed that glitches in infrastructure may be time-draining, and results in abandoned projects.
The research found that 24% of developers spend between 11 and 20 hours every month setting up or altering ML infrastructure. Such tasks need to be completed before any insights from ML can be derived and take up a significant amount of the workday.
For some, the time and resources spent may seem excessive given the limited insights generated, therefore causing nearly half of the respondents to consider ML projects as overly time-intensive.
The issue of excessive time consumption has, according to Civo's findings, led to a considerable number of ML projects being left unfinished. The study reported that only a small 11% of developers have never abandoned a project, with 53% abandoning between 1 and 25% of projects.
Furthermore, 24% admitted to leaving between 26-50% of projects unfinished, and for 8%, the rate of abandonment was even higher, standing at 51-75%.
Nevertheless, Civo's research also uncovered a growing trend of using open-source tools as a potential solution to these hurdles. The survey findings showed that over 73% of respondents found utilising open-source reduced the process time from implementation to insight.
To break it down, 46% saved between 0 to 10 hours, 25% saved 11 to 20 hours, 12% were able to save between 21 to 30 hours and another 12% managed to save over 30 hours.
Civo's Chief Innovation Officer, Josh Mesout, voiced his concerns about the challenges ML developers are facing. He acknowledged the increase in the use of machine learning as a common problem-solving tool.
"We have noticed many developers who are being tasked with deploying ML are not ML experts," Mesout stated. "Instead, they're domain experts in need of the insights and support of ML projects and tooling."
Mesout pointed to a lack of understanding of the potential of open-source tooling, stressing that more needs to be done to highlight their benefits in significantly cutting down on the time and resources spent on ML projects. He believes that with access to open-source, developers can utilise ready-made resources created by ML experts, thus focusing their efforts on generating insights.
Several emerging services can deal with widely recognised pain points of ML. Mesout emphasised that with access to open-source tooling, developers could use ready-made resources by ML experts, thereby centring their time on generating the results they need instead of handling the surrounding infrastructure.